Artificial
synapses copy brain dynamics
By
Kimberly Patch,
Technology Research News
The human brain is a stunningly vast and
complicated network. It is a massively parallel web of about 100 billion
neurons that each harbor about 10,000 connections to other neurons. The
functions the biological brain carries out are even more impressive --
a network of neurons can easily outperform any electronic network of the
same size.
In an attempt to uncover at least some of the brain's computing efficiencies,
a group of researchers from Graz University of Technology in Austria and
Cold Spring Harbor Laboratory have built an artificial neural
network architecture that mimics the way neurons time their signals
to each other.
In going about its routine business, the brain regularly solves difficult
computational problems that remain beyond the reach of the most powerful
computers, said Thomas Natschlager, a research and teaching assistant
at the Institute for Theoretical Computer Science at Graz University of
Technology. The processes the brain uses for doing this, however, have
remained elusive.
One thing the brain can do that is very difficult for computers is react
appropriately to a signal that varies over time, like spoken language.
This is essentially a complicated, or nonlinear filtering function. A
filter is any device that produces output in response to an input signal
over time, and the output of a simple linear filter varies directly with
the signal it's reacting to.
Nonlinear filters, which are more complicated mathematically and thus
more difficult to simulate, are also prevalent in the natural world. The
brain is essentially a big nonlinear filter that perceives input like
auditory and visual signals and produces output like arm movements or
speech, Natschlager said.
Recent research into the dynamics of neurons provided the clue the researchers
needed to work out a neural network architecture that performs more like
the brain. The research shows that neurons gain information from the timing
of electrical signals in ways that electronics-based computers do not.
It is well known that synapses incorporate learning by strengthening and
weakening connections over a period that may encompass several days. In
the case of long-term learning, synapses change in order to store knowledge,
and the changes remain stable for years.
It is also becoming apparent that neurons can also change temporarily
for a few seconds or milliseconds in order to perform a task of the moment.
This ability to reconfigure the network on-the-fly may help neurons increase
their computing power.
"Usually it is assumed that a synapse responds to each incoming input,
[or] spike, with an electrical impulse, [or] postsynaptic potential with
the same amplitude, but this is not true," said Natschlager.
Experimental evidence has shown that the amplitude of the responding electrical
impulse depends on the exact timing of previous inputs. Essentially, the
response of a neuron to a neighboring neuron's signal depends on the history
of multiple signals, or spike trains between them. The synapses between
neurons "are not static devices, but respond differently to each input,"
said Natschlager.
The researchers model incorporates this biological trick, allowing synaptic
strength between neurons to continually change. "The architecture we propose
provides a framework for studying how neural circuits compute in real-time,"
said Natschlager.
The researchers' work added evidence that synaptic dynamics are a key
to the brain's efficiency by showing that a relatively simple artificial
neural network that incorporates dynamic synapses can do some of the difficult
things a brain does.
The dynamic model of timing between neurons gave the network another parameter
to work with, which increased its real-time processing capabilities. The
model allows "rather simple networks to compute an arbitrary nonlinear
filter. Such networks can approximate a surprisingly large class of nonlinear
filters," according to the researchers.
The research is a contribution to understanding how the biological model
of temporarily fluctuating synapse strengths can speed networks, said
Michael Arbib, a professor of computer science and neuroscience at the
University of Southern California. "It's... the latest chapter in the
idea that if you take seriously the rapid changes of the synapses you
can get a lot more computing power in your network," he said.
The sophistication of the brain's network hides a lot of complications
from us, which makes what we do seem easy, Arbib added. For instance,
"the brain doesn't work by... keeping only [like] information together.
It has lots of specialized subsystems. The question is how do those different
subsystems know which of the information they have goes with specific
information that another system is processing. That's called the binding
problem -- how do you bind together these different pieces of information,"
he said.
The Graz and Cold Spring Harbor researchers are working on a new framework
that will allow them to further investigate the types of communications
that can be carried out on spike trains, said Natschlager. "The ultimate
goal is to understand how... neurons and synapses work together to allow
the brain to process time-varying signals so efficiently."
The researchers are also applying their work practically in collaboration
with speech recognition researchers. "We think that within a time frame
of five years we will know how well dynamic synapses are suited for real
world applications," Natschlager said.
Natschlager's research colleagues were Wolfgang Maas of Graz University
of Technology in Austria and Anthony Zador of Cold Spring Harbor Laboratory.
They published the research in the February, 2001 issue of the journal
Network: Computation and Neural Systems. The research was funded by Neurocolt2
and the Austrian Science Fund (FWF).
Timeline: > 5 years
Funding: Government, Institutional
TRN Categories: Neural Networks
Story Type: News
Related Elements: Technical paper, "Efficient Temporal Processing
with Biologically Realistic Dynamic Synapses," Network: Computation and
Neural Systems, February, 2001. The paper is posted at http://www.igi.TUGraz.at/tnatschl/psfiles/dynsyn-preprint.ps.gz
.
Advertisements:
|
June
13/20, 2001
Page
One
Stressed silicon goes
faster
Artificial
synapses copy brain dynamics
DNA device detects
light signals
Lightwaves
channel atoms to make chips
Process promises
better LCD production
News:
Research News Roundup
Research Watch blog
Features:
View from the High Ground Q&A
How It Works
RSS Feeds:
News | Blog
| Books 
Ad links:
Buy an ad link
Advertisements:
|
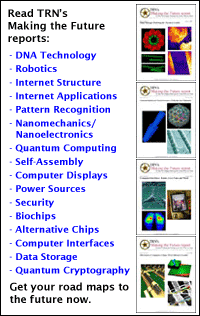
|
|
|