Computers
sort gender in a binary world
By
Ted Smalley Bowen,
Technology Research News
In some respects, computers have helped
mitigate the significance of gender in society. The Internet, for instance,
gives people control over whether to reveal their gender.
At the same time, however, computers can sort people by gender by comparing
faces or voices to a database of features or voice samples. But today's
practical applications have weaknesses. Off-center images of faces can
be hard to interpret, as can crowd shots. Ambient noise can make it difficult
to decipher voice samples.
A group of researchers at The Pennsylvania State University are using
a type of pattern recognition software to determined the gender of both
faces and voices, then merging the data to produce more accurate results.
Support vector machines (SVMs) are a type of computer learning system
that can be trained to screen for certain data in order to make a given
classification. They analyze data by comparing the information to a pair
of previously defined choices, such as the sexes.
SVMs have been used to identify gender using images of faces, but not
voice clips. Today's methods for identifying the gender of voices are
generally less sophisticated than those for facial ID.
To test their scheme, the Penn State researchers trained their system
to screen thumbnail images of faces and voice samples for gender characteristics.
They then presented it with a separate set of pictures and voices, which
the SVMs designated male or female. Finally, they merged the image and
voice results.
The twice-sifted results had a 95-percent accuracy rate, according to
Rajeev Sharma, associate professor of computer science and engineering
at Penn State.
The researchers' multi-modal, multi-stage learning scheme is generic enough
to apply to other decision fusion scenarios as well, said Sharma. "It
involves first building classfiers for each of the two modalities separately,
followed by a separate learning stage in which the fusion of decisions
is learnt. This creates a robust decision fusion from two disparate sources,"
he said.
The researchers have tested the method with head-on, static images and
sound clips that are free of background noise.
The method is fairly easy to implement, according to Sharma. It calls
for basic audio visual equipment and a computer to analyze the data. The
system can handle images that are rotated as much as about 20 degrees,
Sharma said.
To train the face-screening SVM, the researchers used 1,056 facial images
from 600 20- by 20-pixel thumbnail pictures and their mirror images. The
researchers culled the images from several databases.
The group then trained a speech-classifier SVM with 300 voice samples
derived from a spoken alphabet database dictated by 150 male and female
subjects.
The researchers boiled the training material down to 147 image and 147
voice samples. They grouped the results into two-dimensional matrices,
and used 47 of them to train the fusion SVM and 100 for testing it.
A Penn State spin-off venture plans to commercialize the technology for
market research in about six months, according to Sharma.
Eventual uses could include applications that tailor digital content based
on gender in a variety of settings, including information kiosks, according
to Sharma.
The work could potentially have widespread applications, according to
Jeffrey Cohn, associate professor of psychology and psychiatry at the
University of Pittsburgh. "Men's and women's faces differ in both local
features and in shape. The Penn State algorithms appear to capture and
represent [the appropriate] features. They also include vocal parameters
when available. By combining these types of multi-modal data in a classifier,
they potentially can achieve robust discrimination," he said.
At the same time real-world conditions could derail the applications,
Cohn added. "The question is under what range of parameters can the system
perform satisfactorily. Technical challenges include pose, image resolution,
occlusion, number of individuals in the image, and image complexity. Sun
glasses, for instance, foil face recognition algorithms, and may do the
same for gender recognition."
According to Sharma, the system should function well with voice recognition
systems, which require relatively unadulterated sound. The group has yet
to test the system's tolerance of extraneous, ambient noise in real world
situations, he added.
Sharma's research colleagues were Leena Walavalkar and Mohammed Yeasin.
The research was funded by the National Science Foundation and Penn State.
Timeline: Six months
Funding: Government, University
TRN Categories: Pattern Recognition; Computers and Society
Story Type: News
Related Elements: None
Advertisements:
|
January
30, 2002
Page
One
Crystal stores light pulse
Rocket chips
to propel small satellites
Computers
sort gender in a binary world
Quantum network withstands
noise
DNA computer readout glows
News:
Research News Roundup
Research Watch blog
Features:
View from the High Ground Q&A
How It Works
RSS Feeds:
News | Blog
| Books 
Ad links:
Buy an ad link
Advertisements:
|
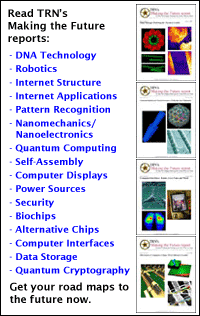
|
|
|