Model keeps virtual eyes right
By
Kimberly Patch,
Technology Research News
You can tell a lot about a person by noticing
where that person's gaze falls, and how long it stays.
Researchers from the University of Southern California have developed
a computer simulation of the areas in the primate brain that perform initial
visual processing, and have used the neurobiological model to produce
realistic automatic head and eye movements in a virtual model of a human
head.
The model shows that the process that drives people to look at
interesting things in a scene is simpler than previously thought. "This
model shows that very basic neural feature detectors may actually explain
a lot of how attention is directed to particular objects in scenes," said
Laurent Itti, an assistant professor of computer science, psychology and
neuroscience at the University of Southern California. Feature detectors
recognize a few simple features, like motion, color, bright spots, and
edges.
The model could be used to study how attention works in a variety
of settings ranging from architecture to graphical user interfaces to
camouflage and surveillance situations, and to improve video compression
algorithms, Itti said.
The model is different from traditional computer vision approaches,
said Itti. Usually an algorithm is designed for a given environment, like
the indoors, a freeway, or Mars, and given targets, like people, cars
or guns, said Itti. "Our approach... makes no such commitment," he said.
"There is no assumption in the design of our model about the type of images
it will process, nor the types of objects it should find interesting."
Instead, the model depends on biology. It commits to looking in
a particular direction depending on which type of visually responsive
neural detectors exhibit an unusual level of activity.
The detectors are modeled after corresponding types of neurons
in the primate brain, said Itti. Such neurons exist in the retina and
in three separate areas of the brain -- the lateral geniculate nucleus
of the thalamus, the primary visual cortex, and the posterior parietal
cortex.
The principal is simple, said Itti. Visual input is analyzed in
parallel by very simple neural detectors, he said. "Each responds to prototypical
image properties like the presence of a red color blob, the presence of
a vertical edge, or the presence of a bright spot on a darker background."
The researchers' model creates feature maps responsible for a
given elementary visual property -- like vertical edges -- over the entire
visual field at a given spatial scale.
The feature maps are endowed with competitive dynamics drawn from
interactions previously discovered in monkey brains. The process dictates
that maps that contain too little activity or too much activity will die
off, while maps that contain a region that has a significantly different
activity level from other regions will be amplified. "As a result, each
feature map will end up highlighting only one or a few regions that are
different from the rest, and thus will tend to attract attention," said
Itti.
A checkerboard that contains a red dot in one of the squares,
for example, excites vertical and horizontal edge detectors at many locations.
Because many of the same types of detectors are excited, they are suppressed.
The red dot excites the red-color detector at only one location, however,
which makes it a strong attention attractor.
All the feature maps are summed up into a saliency map that measures
how conspicuous every location is in a given scene, said Itti. The software
scans the map to choose the most salient target for attention.
Given a scene, the software output is a scanpath, or sequence
of locations the model observes in a scene.
The model is strongly correlated with human scanpaths recorded
from human subjects using an eye-tracking machine.
Before the researchers' model, the guidance of attention towards
interesting objects in a scene was considered a highly cognitive process,
possibly involving internal three-dimensional representations of scenes,
said Itti. The model shows that simple, low-level feature detectors produce
scanpaths similar to those of humans even though there is no form of cognition
in the model, he said.
Given the model's similarity to real human subjects, the researchers'
logical next step was to see whether the model could prove useful in animating
artificial humans, said Itti.
Realistic animation is harder than it seems. The main challenge
in animating a virtual head is to figure out where to point the animation's
gaze. "People are extremely good at judging where another person is looking,"
said Itti. "Any inaccuracy in pointing the gaze of the character towards
objects that a human observer would judge are interesting would be easily
detected by people interacting with the virtual agent," he said.
The researchers' model proved successful at directing gaze, said
Itti.
A second challenge was endowing the model with accurate eye and
head motions. "The mechanistic details of eye and head motion... follow
fairly complex dynamical equations and are driven by complex neural circuits,
not all of which are fully understood," he said.
The researchers used data recorded from monkeys performing a variety
of eye and head movements to drive fairly simple descriptive equations
that came reasonably close to observed motion dynamics, said Itti. As
a target for attention is selected from the saliency map, its coordinates
are passed to the eye/head movement controller, which is in charge of
creating the correct muscle triggers and the eye/head trajectories that
move the animation's gaze towards the selected target.
A third challenge was putting it all together to create realistic
facial animations, said Itti. "When humans move their eyes and head, this
also creates a number of accompanying facial animations, for example lifting
your eyebrows and forehead skin when you look up," he said. The researchers'
three-dimensional face model includes these details to enable frowning,
realistic eye blinks and other facial expressions.
The model is ready for use now, said Itti.
The researchers are currently working to add object recognition
and cognition to the model, said Itti. "The idea is to start departing
from a purely image-based notion of salience and... go towards a mixed
notion that includes not only image properties but also current behavioral
goals," he said. For example, in a situation where a character is driving,
palm trees should be ignored; when the character is counting palm trees,
however, cars should be ignored.
Other possible applications include target detection tasks, in
which the system would automatically pick out salient targets from cluttered
environments. These could include traffic sign and pedestrian detection
in smart car applications, and military vehicle detection, said Itti.
In experimental results using high-resolution rural images, the model
located a target with fewer shifts of gaze than an average of 62 human
observers in 75 percent of the images it was given, he said.
The researchers' ultimate goal is a better comprehension of how
scene understanding works in humans, said Itti.
Itti's research colleagues were N. Dhavale and F. Pighin. The
work was presented at the International Society for Optical Engineering's
International Symposium on Optical Science and Technology in San Diego,
August 3 through 8, 2003. The research was funded by the National Science
Foundation (NSF), the National Eye Institute (NEI), the National Imagery
and Mapping Agency (NIMA), the Zumberge Innovation Research Fund and the
U.S. Army.
Timeline: Now
Funding: Government; Institute
TRN Categories: Data Representation and Simulation; Human-Computer
Interaction
Story Type: News
Related Elements: Technical paper, "Realistic Avatar Eye
and Head Animation Using a Neurobiological Model of Visual Attention,"
International Society for Optical Engineering's International Symposium
on Optical Science and Technology, August 3-8, 2003, and posted at www.ict.usc.edu/publications/Itti_etal03spienn.pdf
Advertisements:
|
February 25/March 3, 2004
Page
One
Ethanol yields hydrogen
Biochip makes droplet
test tubes
Model keeps virtual
eyes right
Simple optics make
quantum relay
Briefs:
Hot tip boosts
disk capacity
Nanotubes boost
shape recovery
Nanowires spot DNA
mutation
Scans pick
up object orientation
Nanotube mix
makes liquid crystal
Film promises
terabit storage
News:
Research News Roundup
Research Watch blog
Features:
View from the High Ground Q&A
How It Works
RSS Feeds:
News | Blog
| Books 
Ad links:
Buy an ad link
Advertisements:
|
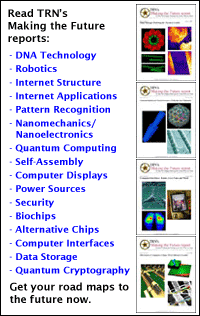
|
|
|