Recommenders can skew results
By
Kimberly Patch,
Technology Research News
Just how accurate are the recommender systems
online media sellers use to allow buyers to pass on their judgments about
books, movies and CDs to their fellow consumers?
Researchers from the University of Minnesota have shown that the
way recommender systems are set up can affect the opinions they evoke,
and that artificially high or low recommendations can raise or lower subsequent
recommendations.
Displaying a prediction introduces bias, said Joseph Konstan,
an associate professor of computer science and engineering at the University
of Minnesota. "Lying by [skewing rankings] higher or lower... biases the
subsequent rating in that direction," he said. "Even the 'correct' rating
led people to select that value more often."
The distortion this chain of events induces may influence consumer
buying in the short-term, but adversely affects long-term consumer trust
in the system, said Konstan. "While a system can get away with a small
degree of lying... in the long run dishonesty erodes trust and satisfaction,"
he said.
The researchers' work is consistent with a long line of psychology
studies showing that people shift opinions to conform to a group, said
Konstan. "There's a bunch of psychology research that suggests that people
exhibit a desire to conform," he said.
In a 1969 study published in Sociometry, for instance, a research
team headed by Serge Moscovici found that about a third of the people
in a group would call a blue block green if the researchers planted a
couple of vocal people in the group who called the block the wrong color.
The Minnesota researchers conducted three experiments with a total
of 536 people in order to see how previous ratings affected the test subjects'
recommendations.
They used the Movie Lens recommender system, which includes about
70,000 users, 5,600 movies and around 7 million ratings.
In the first experiment, the researchers asked participants to
rate 40 movies the participants had previously rated. The experiment presented
lists of 10 movies using four different recommender configurations. The
participants used a 1- to 5-star rating scale. One configuration showed
no predictions, the second showed predictions equal to the user's original
rating, the third showed predictions one star above the original rating,
and the fourth showed predictions one star below the original rating.
The results revealed that people were fairly consistent in re-rating
movies when there were no other ratings on-screen. Participants gave the
movies the same ratings 60 percent of the time, one star below the original
rating 20 percent of the time and one star above the original rating 20
percent of the time.
The results also showed that having other ratings on screen, whether
they matched the user's original rating or were one star up or down, influenced
the second rating the user gave. When ratings were bumped up or down one
star, participants rated nearly 30 percent of movies one star above or
below the original rating, respectively.
In the second experiment, a group of people rated 48 movies for
the first time. The researchers predicted what people's ratings would
be, then added or deleted stars in the same way as in the first experiment.
They then repeated the experiment with a control group without manipulating
the ratings shown to the participants.
The users were again swayed by incorrect ratings. In addition,
those shown incorrect ratings were more dissatisfied with the process
than the control group, probably because they sensed that the predictions
were inaccurate, according to Konstan.
Other research shows that people treat computers socially, similarly
to the way they treat other people, said Konstan. "We speculate that this
effect may be skewing ratings towards the computer-displayed prediction,"
he said.
The research did not distinguish between the users' actual preferences
and the ratings they entered, said Konstan. "We do not know whether [the
rating system] really changes the persons' preference, or just the rating
they choose to enter," he said.
Following up on their 1969 experiment, Moscovici's group looked
at people's actual preferences in addition to what they said, and showed
that even those who did not call the blue block green rated blue-green
slides as more green than pretests predicted they would. The researchers
produced similar results after going a step further by asking participants
to rate the color of the afterimage they saw after looking at the slide.
Afterimages are involuntary artifacts manufactured by the human visual
system.
The Minnesota study confirms the line of research that shows that
people tend to conform with suggestions, and points out that care is needed
to avoid introducing biases in information interfaces, said Konstan.
In a third experiment, the researchers asked users to rate three
sets of 15 movies they had previously rated using three different scales:
thumbs up or thumbs down, a scale from -3 to +3 not including a zero,
or a 0.5 to five-star scale in half-star increments.
This experiment showed that people prefer finer-grained scales,
and that finer-grained scales are ultimately more accurate. Participants
rated the half-star scale the most satisfactory followed by the plus or
minus three scale, and were least satisfied with the binary scale. The
finer-grained scales are more accurate because people tend to give borderline
movies the benefit of the doubt when forced to rate on a coarse scale,
according to Konstan.
To evoke recommendations that are as independent as possible,
recommender systems should give consumers an environment that allows them
to provide ratings without having to see previous ratings, Konstan said.
And the system should provide fine-grained rating scales rather than simpler
thumbs up, thumbs down ratings, he said.
The Minnesota researchers are ultimately aiming to better understand
how interfaces, social and economic structures, and other design factors
influence people's participation in and use of recommender systems, said
Konstan. The design implications of the current results can be used immediately
to improve recommender sites, he said.
Konstan's research colleagues were Shyong K. Lam, Istvan Albert
and John Riedl. They presented the results at the Association of Computing
Machinery (ACM) Computer-Human Interaction conference held in Fort Lauderdale,
Florida April 5-10, 2003. The research was funded by the National Science
Foundation (NSF).
Timeline: Now
Funding: Government
TRN Categories: Internet
Story Type: News
Related Elements: Technical paper, "Good Ratings Gone Bad:
Study Shows Recommender Systems Can Manipulate Users' Opinions," presented
at the Association of Computing Machinery Computer-Human Interaction (ACM-CHI)
conference, Fort Lauderdale, Florida, April 5-10, 2003; "Influences of
a Consistent Minority on the Responses of a Majority in a Color Perception
Task," Sociometry 32 Moscovisi & Personnaz, 1980.
Advertisements:
|
July 2/9, 2003
Page
One
DNA makes nano barcode
Study reveals Net's parts
Recommenders can skew
results
Light pipes track motion
News briefs:
Material helps
bits beat heat
Process puts
nanotubes in place
Printing method
makes biochips
Tiny T splits light
Tiny walls sprout
nanowires
Big sites hoard links
News:
Research News Roundup
Research Watch blog
Features:
View from the High Ground Q&A
How It Works
RSS Feeds:
News | Blog
| Books 
Ad links:
Buy an ad link
Advertisements:
|
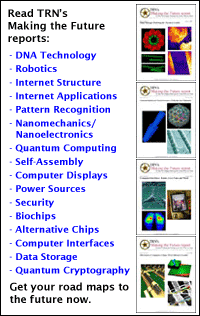
|
|
|