Search
method melds results
By
Chhavi Sachdev,
Technology Research News
Search for the term ‘heartburn’ in a medical
database and a typical search engine will spit out a long list of documents
that contain the term along with a brief summary of each hit. Researchers
at Columbia University have come up with an automated engine that promises
to take the search a step further by comparing the returns.
The engine finds a set of documents, defines the query term in natural
sentences using text pulled from those documents, and summarizes their
hyperlinks, allowing the user to see both the information present in a
set of documents and what is unique to each hit, according Min-Yen Kan,
a computer science researcher at Columbia.
A summary from the heartburn search, for instance, would describe the
causes, symptoms and prognosis of the malady, he said.
The query results also include details like relative length that differ
among types of documents. A 10-page article about heart disease might
be considered long, for instance, but a 100-page novel is rather short.
The results also detail whether or not a document in the set has a diagnosis
section, or which documents contain figures and tables.
In order to compare results, the system sets up a topic tree for every
query term by comparing all the subheads in the documents. “Each document
is viewed as a tree of topics and these trees are intersected to find
commonalties and differences,” said Kan.
The definition, symptoms, and causes of most problems are nearly always
signaled by distinct section headings within documents. Each of these
branches could have five sub-branches.
“Once a search engine knows that the document is relevant to the search
query... the system matches the query to a particular node of each document,”
said Kan. The node might be the root node of the tree, as in the case
of ‘heartburn treatments’, or somewhere in the middle, as in the case
of ‘facts about heartburn,’ he said.
The system sets up a composite tree by comparing all the documents to
get an idea of what an average document is like. Even if the heartburn
documents don't have ‘prognosis’ as a topic, the system can infer the
typical structure of patient information documents by looking at related
documents that have the same structure, Kan said.
The software sorts references and subheads, focusing on those that occur
more often in the query documents. When a section is not featured in another
document and is too far down from the main branches, it might be discarded
as irrelevant or too intricate, said Kan.
It generates natural language summaries of text based on the types of
information that are really useful, Kan said. It extracts sentences or
list items that occur across documents and checks morphological and subject-verb
agreements; then it adds words to make clear sentences, he said.
The software also extracts descriptors such as the author’s name, media
format, and categorization keywords if they are available as metadata
in the documents.
The researchers can change the parameters of a search to trade speed for
accuracy; the running time for the system to summarize five documents
can vary from a few seconds to a few minutes, according to Kan.
The system is currently very accurate, he said, “but this is because we
have been working only on developing the system using a closed set of
example documents. As we expand the types of documents we will handle,
more noise and error will be introduced, reducing the accuracy.”
The medical documents the Centrifuser project draws from are very similar
in domain and genre. The software could easily be adapted to other sets
of documents grouped by genre in achival repositories or intranets, Kan
said.
Current document summarization techniques are not particularly satisfactory,
said Justin Zobel, an assistant professor of computer science at RMIT
University in Australia. “Developing a careful architecture for summarization,
as in this work, is definitely a good way to proceed,” and including indicative
summaries is also helpful, he said.
The Columbia engine is still in an early phase, however, Zobel added.
“The system is not yet at the stage at which realistic user studies can
be done.”
The researchers plan next to evaluate the current system and base future
development according to users’ criticisms, Kan said. They also plan to
make the topic-tree indexing software better. The system could be in use
in 5 to 7 years.
Kan’s research colleagues were Kathleen R. McKeown and Judith L. Klavans.
They presented the research at the 8th European Workshop on Natural Language
Generation in Toulouse, France, July 2001. The research was funded by
the Digital Libraries 2 Initiative of the National Science Foundation
(NSF).
Timeline: 5-7 years
Funding: Government
TRN Categories: Natural Language Processing; Databases and
Information Retrieval
Story Type: News
Related Elements: Technical paper, "Applying Natural Language
Generation to Indicative Summarization," presented, held on 6 7 July,
2001. Posted on the Computing Research Repository on July 16, 2001 at
http://xxx.lanl.gov/abs/cs.CL/0107019
http://www.cs.columbia.edu/~min/research/centrifuser/wnlg01/wnlg01.pdf
Advertisements:
|
January
9, 2002
Page
One
Sunlight turns water
to fuel
Search method melds results
VR tool keeps
line of sight in hand
Laser pulse penetrates
glass
Model tracks desert spread
News:
Research News Roundup
Research Watch blog
Features:
View from the High Ground Q&A
How It Works
RSS Feeds:
News | Blog
| Books 
Ad links:
Buy an ad link
Advertisements:
|
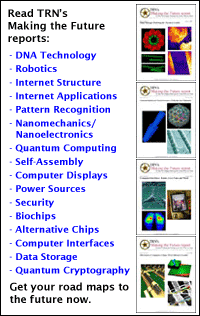
|
|
|