Online popularity tracked
By
Eric Smalley and Kimberly Patch,
Technology Research News
How do you measure the popularity of items
available for download or sale on the Internet?
Researchers from Cornell University and the Internet Archive have
devised a way to measure users' reactions to an item description: a batting
average of the number of users who go on to download the item divided
by the number of users who read the description. This mirrors the traditional
baseball batting average of the ratio of a player's hits to at bats.
The item description batting average is different from just tracking
the output of a hit counter, which measures the raw number of item visits
or downloads, said Jon Kleinberg, an associate professor of computer science
at Cornell University. "The batting average addresses the more subtle
notion of users' reactions to the item description as it appears in the
fraction of users who go on to download the item."
A users' batting average reveals something about the nature of
on-line popularity, can make users explicitly aware of shifts in popularity,
and allows administrators of large sites to quickly identify sudden and
potentially significant effects on the popularity of particular items
and prepare accordingly.
The researchers found that on the Web, popularity often changes
abruptly rather than gradually. "For example, an item would be getting
downloaded at a rate of roughly 38 percent, and then at exactly 8: 35
a.m. on February 20, it would drop to about 24 percent and stay there
for the next several days," said Kleinberg.
Although the abrupt shifts were initially surprising, "the underlying
reason is intuitive," said Kleinberg. "Your popularity on the Web is affected
by having a high-traffic site decide to link to you or mention you in
some way and this link or mention is added at a precise moment in time,"
he said.
This draws a lot of traffic to the item's description, and the
traffic is "a new, larger mix of users with a possibly different set of
interests than the niche population that has been viewing it up until
then," said Kleinberg. This can either drive the batting average up abruptly
if this larger population decided that they really liked the item, or
down if, by and large, they did not, he said.
In working with data from the Internet Archive, which maintains
a digital collection of publicly available films, concerts and books,
the researchers found that abrupt shifts corresponded closely to real-world
events that drove what was often a new mix of users to view an item's
description.
Analyzing item popularity dynamics at a given Web site can help
characterize the impact of a range of events taking place both on and
off the site, according to Kleinberg. The batting average shows a change
in the make-up of the population, as reflected in the fraction that was
interested in downloading the item, he said.
A practical benefit of the batting average is making users aware
of popularity shifts, said Kleinberg. "For each item, we can imagine keeping
a running history of the on-site spotlighting and active external links
that have affected the item over the previous years and months, together
with a summary of the effect on the item's popularity," he said.
The same goes for reviews of items, said Kleinberg. "Since the
appearance of a strong positive or negative review can affect the batting
average, there's the intriguing possibility of creating a quantitative
measure of 'review impact'."
The researchers tracked abrupt shifts in batting averages using
an algorithm based on Hidden Markov Models, a type of pattern recognition
algorithm that observes a sequence of states in order to identify the
system producing them and make predictions about future states. Hidden
Markov Models are widely used in speech recognition software; a spoken
word is the system and the sounds that make up the word -- phonemes --
are the states.
"In this case, the hidden states correspond to the possible values
of the current batting average for the item, and so we can analyze the
sequence of item downloads to estimate the most likely moments at which
this batting average changed," said Kleinberg.
The researchers are working on models that will be able to infer
what a user is doing and what a user is trying to accomplish when visiting
a site like Amazon, arxiv.org, or the Internet Archive. "The batting average
and its analysis through Hidden Markov Models is a simple example of such
a model, but richer models might allow us to guess that one user is lost
and not sure of what to purchase, while another is in the process of seeking
a specific item," said Kleinberg.
Applications based on the researchers' current method are possible
in the near-term; better models that can infer what a user is doing are
several years out, said Kleinberg.
Kleinberg's research colleagues were Jonathan Aizen of the Internet
Archive and Daniel Huttenlocher and Antal Novak of Cornell University.
The work appeared in the January 6, 2004 issue of the Proceedings of
the National Academy Of Sciences. The research was funded by the National
Science Foundation (NSF) and the David and Lucile Packard Foundation.
Timeline: > 1 year; 3 years
Funding: Government; Private
TRN Categories: Internet
Story Type: News
Related Elements: Technical paper, "Traffic-Based Feedback
on the Web," Proceedings of the National Academy Of Sciences, January
6, 2004.
Advertisements:
|
July 28/August 4, 2004
Page
One
Photonic chips go 3D
Online popularity tracked
Summarizer gets the idea
Electric fields assemble
devices
Briefs:
Process prints
silicon on plastic
Tool automates
photomontage edits
Device promises
microwave surgery
Hologram makes
fast laser tweezer
Chemistry yields
DNA fossils
Particle
chains make quantum wires
News:
Research News Roundup
Research Watch blog
Features:
View from the High Ground Q&A
How It Works
RSS Feeds:
News | Blog
| Books 
Ad links:
Buy an ad link
Advertisements:
|
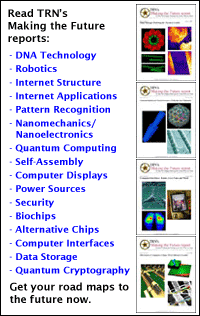
|
|
|